Automate Your RFP Response Process: Generate Winning Proposals in Minutes with AI-Powered Precision (Get started for free)
7 Critical Metrics That Define SaaS Support Hours Effectiveness in 2024
7 Critical Metrics That Define SaaS Support Hours Effectiveness in 2024 - First Response Time Under 5 Minutes Through AI Powered Tagging
In today's SaaS landscape, a first response time (FRT) of under five minutes is no longer a luxury but a necessity. Customers anticipate quick interactions, and failing to deliver can damage their perception of your service. AI-driven tagging systems are a key tool in achieving this goal. They can rapidly sort through the influx of support requests, helping your teams pinpoint the core issue and respond with speed. We see an average FRT across the industry hovering around 12 hours, a stark contrast to the 5-minute expectation most customers have. AI has the potential to bridge this gap, significantly lowering response times, even down to seconds in some cases. Given the strong connection between speed of response and customer happiness, companies who don't embrace these AI tools risk losing ground in an ever-competitive market. Ignoring the urgency of this need could have long-term ramifications.
The typical first response from a human support agent is often frustratingly slow, frequently exceeding ten minutes. However, AI-driven tagging systems show promise in slashing that time to under five minutes. The approach relies on instantly classifying support tickets based on keywords or phrases within the request, allowing for a rapid initial response.
It's interesting that research indicates AI-powered tagging leads to around a 30% faster resolution overall compared to traditional routing. While this seems significant, the practical impact of that speedup might vary wildly depending on the specifics of a support system's workflow. This begs the question of how much real-world benefit a faster ticket resolution actually produces, particularly in contexts where human intervention is needed for more complex problems.
The foundation of this kind of AI tagging lies in natural language processing. These systems don't rely on predefined categories, instead learning to parse requests in real-time, attempting to decipher the intent behind a support query. The systems can then process thousands of requests concurrently, spotting critical issues and routing them instantly to the appropriate teams. That's potentially useful in reducing the strain on support staff needing to manually triage problems.
However, the underlying AI models are reliant on the quality and quantity of their training data. Over time, they presumably get better at tagging through continuous learning, which can lead to gradual efficiency gains. The system ideally adapts to how customers actually express their needs, even accommodating newly coined phrases and slang that traditional systems might miss.
Additionally, these systems can be insightful beyond immediate responses. By analyzing the frequency of specific tagged issues, it might expose recurring problems within a product or service. This allows for proactive adjustments or feature enhancements, leading to overall improved product quality.
In some ways, the effect is similar to an automated escalation path, freeing human agents to focus on more demanding issues. Studies suggest that implementing AI tagging can shrink average handle times by a quarter. This efficiency can be valuable, but it could also lead to potential drawbacks if a support team is left understaffed relative to the demands placed upon it.
The AI systems don't get stuck relying on outdated, static support databases. Instead, their ability to adapt and update their tagging criteria in real-time ensures that classification remains accurate and effective. This flexible approach is likely a significant advantage for systems facing continuous change in user needs.
Ultimately, the goal is to make customers feel heard and understood, which is often intrinsically tied to speed. Support teams that achieve sub-five-minute response times using these approaches have seen promising increases in customer satisfaction and loyalty. Yet, whether this always translates to significant gains in customer lifetime value and business success remains a subject for ongoing investigation.
7 Critical Metrics That Define SaaS Support Hours Effectiveness in 2024 - Monthly Cost Per Ticket Below $8 Using Automation Workflows
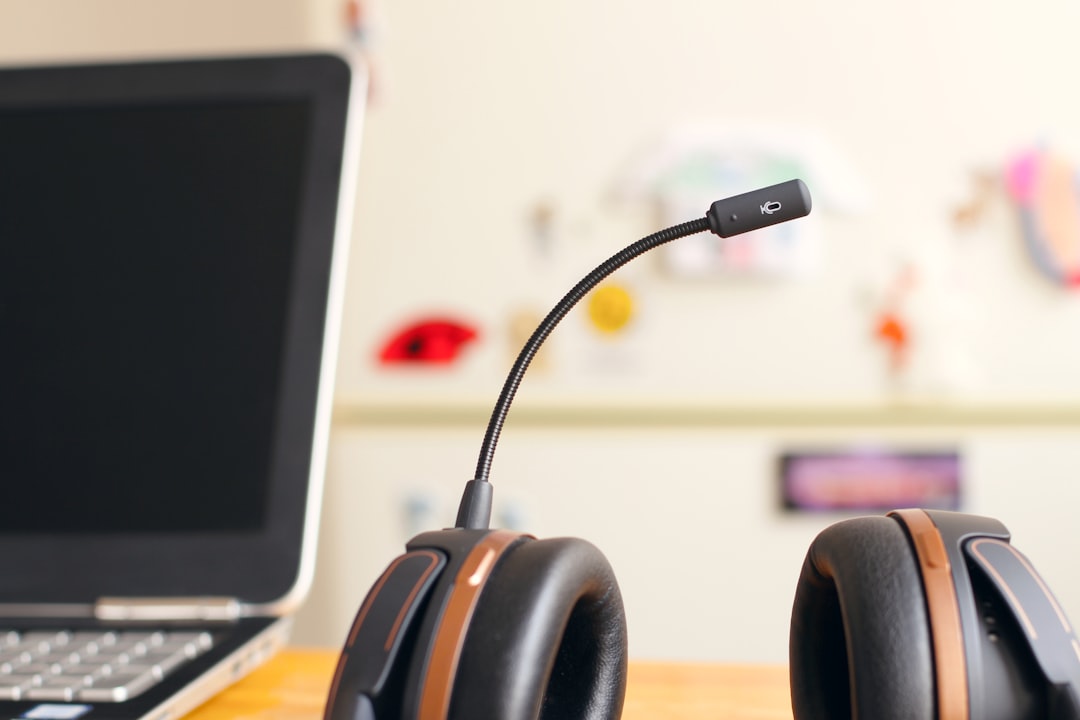
Within the evolving SaaS support landscape of 2024, achieving a monthly cost per ticket below $8 is a realistic goal, largely due to advancements in automation workflows. Automation tools, such as chatbots and automated ticket routing, have the capacity to streamline operations by decreasing manual effort. This reduction in human intervention can translate directly into a lower cost per ticket. However, achieving cost efficiencies shouldn't compromise the quality of the customer experience. Companies must carefully manage the balance between automation and maintaining a high level of customer service.
It's important to acknowledge that while automating certain aspects of support can reduce ticket volume and potentially lead to more complex issues being handled by remaining human agents, it also raises questions about staffing levels. Could automation lead to a situation where the remaining staff are overburdened or face challenges in maintaining morale and engagement with more demanding, complex tasks?
As SaaS companies strive for greater support efficiency and cost optimization, understanding the implications of cost-per-ticket metrics becomes increasingly vital. Companies must analyze how ticket costs impact overall support effectiveness and how this translates into the long-term health and success of their support operation.
Thinking about the cost of handling each support ticket is a fundamental part of understanding how efficient a SaaS company's support team is. This metric often shifts as companies adopt things like self-service tools and automated workflows, such as chatbots.
When you use automation to handle a lot of the common support requests, you can see a reduction in the overall number of tickets that need a human to deal with. This in turn can lower the cost per ticket because you're dealing with fewer, but often more complex, issues, resulting in a smaller workload for the support team.
It's important to strike a good balance between keeping the cost of handling tickets low and maintaining a high level of customer satisfaction. If you optimize too heavily for cost, you might sacrifice customer experience, and that's detrimental to growth.
It's fascinating to see how different automation approaches impact the cost. Industry averages for cost per ticket can fluctuate dramatically, ranging from really low-cost, self-service options that might be a fraction of a dollar per ticket to high-cost walk-up support scenarios that can cost hundreds of dollars per ticket. This variation seems tied to the complexity of issues and the type of support needed (like, Level 3 support with on-site technicians often pushes costs higher, potentially up to $100 per ticket).
Understanding the true cost of support tickets, including the impact of inefficiency, is crucial. It can reveal where support operations might be leaking productivity and possibly exceeding the direct costs involved in keeping the support team running. This type of analysis can point to areas where automation might yield the biggest improvements.
Focusing on things like the cost of acquiring new customers and the number of unique visitors per month helps SaaS companies better understand their financial health and growth trajectory. But it's worth noting that the cost of support and efficiency in that department is often overlooked, despite playing a critical role in the long-term success of a SaaS product. For instance, a subpar support experience can quickly lead to customer churn, wiping out the gains from new customer acquisition. And while keeping the cost of support low is important, it's important to consider that cost is not everything. Investing in a good support experience, even if it costs slightly more in the short term, is often the key to unlocking higher customer lifetime value.
7 Critical Metrics That Define SaaS Support Hours Effectiveness in 2024 - Support Team Utilization Rate at 85% With Smart Queue Management
Striving for an 85% support team utilization rate indicates a well-managed support system, showcasing the delicate balance between using resources wisely and ensuring quick responses. This rate is a strong indicator that smart queue management is working effectively. Smart queue systems help distribute tickets more evenly and reduce delays in handling them. These intelligent systems allow your support team to concentrate on the most important requests, leading to better customer experiences and a more efficient operation. It's crucial to understand, however, that a high utilization rate doesn't automatically mean excellent support or happy support staff. It's a balancing act to achieve high utilization without sacrificing service quality or the well-being of the support team in the long run. We must consider the larger impact and potential downsides before solely chasing high utilization numbers.
When we look at how effectively a support team is used, aiming for an 85% utilization rate is often seen as a sweet spot. This means that, ideally, team members are actively engaged with customer issues 85% of the time they're on the clock. However, it's crucial to keep in mind that constantly pushing for this level can potentially lead to employees feeling burnt out. Finding that right balance is vital to avoid a situation where staff are perpetually overloaded.
If a team is consistently operating at 85% utilization, it often suggests that they're working close to their maximum capacity. Any unexpected surge in support requests can quickly create bottlenecks, causing delays in getting back to customers and resolving their issues. Essentially, while the goal is to maximize use, teams can be too efficiently used.
Smart queue management systems come into play here. They can greatly improve how teams utilize their time by intelligently routing tickets to the agent who is best suited to deal with the issue and who has the most available time. This contrasts with the old, simpler method of just handling requests on a first-come, first-served basis.
It's often observed that a team with a well-managed utilization rate has a positive impact on customer satisfaction. But there's a point of diminishing returns. If a support team is regularly exceeding that 85% target, customer service can start to suffer as the agents get overloaded.
With smarter queue management, agents can focus on specific types of requests. This often means issues get solved faster, which can be great for both the customer and the agent. This specialization also tends to be a plus for agents in terms of job satisfaction and career growth, but only if the overall workload is being carefully managed.
An efficiently utilized support team isn't just reacting to problems; it can also look at patterns in tickets and proactively address potential issues before they become major problems for customers. This is a shift from firefighting to anticipating and preventing problems, which can contribute to a long-term decrease in the overall number of tickets.
However, this close monitoring of a support team can impact the work culture. It can foster a sense of responsibility and accountability but also lead to a culture where agents feel too pressured to constantly meet those metrics. It's important to avoid creating a stifling atmosphere where people are afraid to think outside the box for fear of missing a utilization target.
Even with an 85% utilization rate, there can be hidden inefficiencies. For example, if agents frequently have to switch between tasks or perform redundant steps, the system isn't as smooth as it could be. Teams need to look at their workflow and identify these types of hidden problems to improve performance even further.
As technology in the field of customer service changes, the same utilization targets may need to be revisited. Support teams need to be adaptable because a fixed 85% utilization might hinder growth if customer demands increase and more resources are needed to meet them.
While aiming for an 85% utilization rate may seem like a good way to save money, it's important to also think about the long-term effects on employee well-being and customer experience. Support teams should be thinking about how to support their own staff and the technology they use to maintain a sustainable support structure that can adapt to a changing environment. This is likely to be more beneficial in the long run.
7 Critical Metrics That Define SaaS Support Hours Effectiveness in 2024 - Customer Satisfaction Score Above 95% Through Real Time Feedback
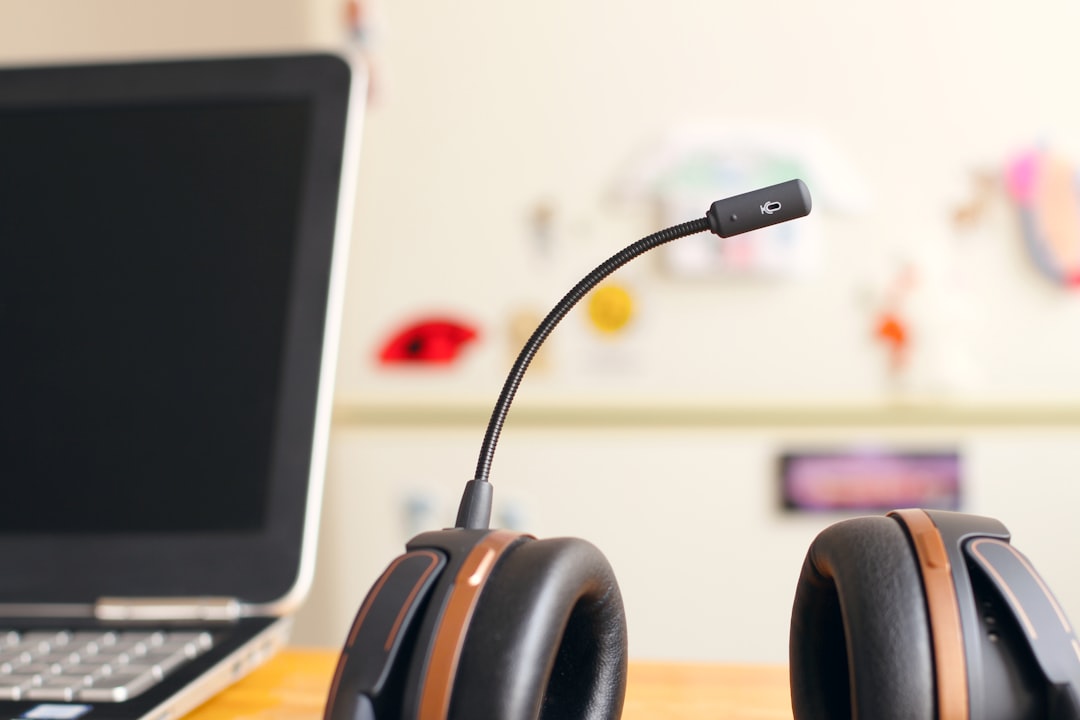
A customer satisfaction score (CSAT) exceeding 95% is a strong indicator of exceptional customer happiness within a SaaS environment. This level of satisfaction often comes from actively seeking out customer feedback in real-time. When companies are responsive and adapt quickly based on direct input, it makes customers feel valued and heard, leading to improved interactions. Real-time feedback gives you a clear window into what's working well and where there are potential pain points, helping your team take action to improve the experience.
Maintaining such a high CSAT is definitely not easy. It necessitates a continued commitment to providing excellent service and addressing concerns rapidly. However, this is critical, as actively measuring and tracking CSAT is vital for SaaS companies to differentiate themselves from the competition and cultivate a loyal user base over the long term. While a high CSAT can be a testament to a good product or service, it’s important to remember that the pursuit of this metric shouldn't come at the expense of employee well-being or the overall sustainability of the support structure. Striving for high scores while remaining conscious of the broader impact is essential.
Customer satisfaction scores (CSAT) above 95% are a strong signal of exceptional customer happiness. While a CSAT of 75-85% is often considered good across many industries, reaching the 95% mark implies a truly remarkable level of satisfaction with a product or service. In the world of SaaS, hitting such a high level is potentially tied to using real-time feedback systems. These tools allow for immediate insights into customer experiences, paving the way for swift adjustments to support strategies. It's interesting that companies with consistently high CSAT scores, like 95% or above, frequently report substantially higher customer retention rates compared to others in their field. It's a compelling idea that happier customers are more likely to stick around.
A strong link between CSAT and revenue growth has been observed. The theory is that each 1% jump in CSAT can potentially correspond to a 5% increase in revenue. This boost likely comes from increased repeat business and word-of-mouth referrals. However, one wonders how accurately we can always trace revenue increases to changes in CSAT, given the many other variables that impact business.
It seems that the ability to capture feedback in real-time helps businesses respond more quickly to customer concerns. Those using real-time feedback systems often see issue resolution times that are about 50% faster compared to businesses relying on more conventional methods like email or surveys. Naturally, getting answers fast leads to better experiences, and those positive experiences might be reflected in those sky-high CSAT scores.
An intriguing feature of real-time feedback is the potential to incorporate predictive analytics. By continuously collecting data about how customers interact with a product, the systems can start to anticipate future needs and problems. Potentially, this allows companies to tweak their support strategies to be more preventative, aiming to avoid customer dissatisfaction before it happens. However, it is also important to understand the data collection process and understand its limitations and biases.
The relationship between customer happiness and employee satisfaction is worth noting. Teams that regularly encounter high CSAT scores often report a sense of greater job fulfillment. Seeing the direct impact of their efforts can be motivating and enhance morale. However, this positive correlation could be a complex chicken-and-egg scenario where high-performing, well-motivated teams result in high CSAT scores, or perhaps it's the other way around.
There's a potential cost benefit to achieving consistently high CSAT, at least in terms of ticket volume. The idea is that fewer customers are calling in with repeated or similar issues, which can improve overall efficiency and decrease support costs. A common estimate suggests that companies with high CSAT can potentially reduce support costs by around 20%. However, the initial investment required for setting up a real-time feedback system might need to be carefully considered in the cost-benefit equation.
The trend is to incorporate multiple channels for gathering feedback, from social media to email. Companies achieving those high CSAT scores often have a diversified approach to collecting information from various customer touchpoints. This multi-pronged approach leads to a more complete understanding of customer preferences and potential areas for improvement.
High CSAT scores are commonly paired with rapid response times. Companies with efficient real-time feedback systems frequently manage response times that are well below two minutes. Again, this speed helps foster a feeling of being heard and cared for, boosting satisfaction. Yet, one might question if the speed of response is a reliable indicator of overall support quality, or if it's just an illusion of attentiveness.
The idea of a continuous improvement loop is important here. High CSAT isn't a static achievement. By analyzing the feedback data, companies can pinpoint problems, adjust their approach, and then measure if these changes lead to improvements in CSAT. Essentially, it's a feedback loop in itself, where feedback itself fuels continuous adjustments and optimization to keep CSAT high. It begs the question of how much feedback is too much and what are the best strategies for incorporating feedback into product and support changes.
In conclusion, CSAT scores above 95% represent a strong indicator of customer happiness and can be linked to positive business outcomes. Real-time feedback systems seem to be a key tool in reaching and maintaining these high levels of satisfaction, leading to faster resolution times, greater retention, and potentially lower support costs. While the benefits appear significant, ongoing research is needed to fully understand the complexities of the relationships between these factors and how to best manage these systems to optimize the entire customer journey.
7 Critical Metrics That Define SaaS Support Hours Effectiveness in 2024 - Average Resolution Time Under 2 Hours Via Knowledge Base Integration
In the SaaS world of 2024, achieving an average resolution time (ART) under two hours is becoming increasingly important, particularly with the help of knowledge bases. This goal underscores a wider trend in customer support: the expectation for incredibly fast solutions. By making a knowledge base readily available, both support agents and customers have a central resource to find answers quickly. This can significantly cut down on the time it takes to address support requests. However, this push for speed shouldn't come at the cost of high-quality support. There's a concern that constantly striving for faster resolution times could negatively affect support quality, potentially leading to staff burnout and a decline in the overall customer experience. Striking the right balance between efficiency and service excellence will be a key challenge for SaaS companies moving forward if they hope to sustain success.
In the realm of SaaS support, achieving a swift resolution to customer issues is paramount. One metric that's gaining traction is the average resolution time (ART), and specifically, keeping it under two hours through the integration of knowledge bases.
It's becoming increasingly apparent that knowledge bases can dramatically accelerate the resolution process, potentially cutting resolution times by up to 70% compared to traditional methods. This efficiency boost has a direct impact on how happy customers are with the support they receive. We're seeing a trend towards self-service solutions where customers are taking the reins to solve issues themselves. Studies suggest that roughly two-thirds of customers actually prefer resolving issues on their own rather than contacting support. It seems that customers increasingly value the autonomy of being able to find solutions quickly, suggesting that knowledge base integration aligns well with evolving customer preferences.
Interestingly, newer knowledge base technologies leverage machine learning algorithms to understand the context of customer queries. This contextual understanding enables more personalized support pathways, which, in turn, improves the accuracy and speed of the response. This level of intelligence moves beyond the limitations of simple, static knowledge repositories. In the context of resource allocation, knowledge bases allow support teams to focus on more complex problems that require human intervention. Routine inquiries are effectively handled by the knowledge base, freeing up valuable time for agents to deal with situations that genuinely need a human touch.
What's really fascinating is that these knowledge bases can continuously refine their content through feedback and historical data. This creates a continuous learning loop, meaning the information within the knowledge base adapts to how customers actually interact with the system. This dynamic approach keeps the knowledge base current and improves the support experience over time.
There's also a clear link between resolution time and customer retention. The faster an issue is resolved, the more likely it seems a customer will stick with a service. Research indicates that companies maintaining a sub-two-hour average resolution time retain up to 10% more customers than those whose average exceeds that threshold. That's a significant impact for businesses, suggesting that investing in fast resolutions can have a tangible impact on the bottom line.
As companies scale their operations, maintaining quick resolution times can be a challenge. However, a well-designed knowledge base makes scaling support more manageable. The ability to efficiently handle a higher volume of tickets without sacrificing speed makes it a key asset for growing SaaS companies. It's also interesting to note that a good knowledge base can deliver a consistent experience across various support channels, such as chat, email, or phone calls. This uniformity across different channels helps build trust with customers, who know they'll receive the same quality of information no matter how they get in touch with support.
The improvements in resolution times also impact the culture of a support team. Achieving faster resolutions fosters a customer-centric mindset within the organization. Teams become more focused on delivering exceptional service, leading to continuous improvements in their workflows and a heightened level of engagement amongst staff.
Finally, tracking average resolution times against industry norms allows companies to benchmark their performance. Achieving a resolution time under two hours doesn't just mean offering good customer support, it positions the company as a leader in the field. In a competitive market, this ability to deliver a high-quality, fast support experience can be a powerful selling point.
All of these observations reinforce the significance of knowledge base integration for boosting the efficiency and quality of SaaS customer support. It's clear that investing in a robust and intelligent knowledge base is no longer an option, but rather a necessity in the evolving landscape of SaaS support.
7 Critical Metrics That Define SaaS Support Hours Effectiveness in 2024 - Support Ticket Deflection Rate at 40% Using Self Service Portals
In the current SaaS landscape, aiming for a 40% support ticket deflection rate using self-service portals is becoming a crucial aspect of effective support. By empowering users to resolve common issues independently, these portals significantly reduce the volume of tickets requiring human intervention. This showcases the importance of prioritizing self-service solutions, a strategy aligned with many customers' desire for greater autonomy in handling their own issues.
Monitoring key indicators, like how often customers choose to use self-service options and how helpful they find the provided information, is vital for making ongoing improvements. Regularly analyzing these metrics helps ensure that the knowledge base and support resources are as relevant and useful as possible. While effectively deflecting a significant portion of tickets can reduce strain on support teams, it's important to recognize that the effectiveness of a self-service portal is intrinsically tied to how readily customers adopt and benefit from it.
The advantages of this approach extend beyond efficiency gains, positively influencing customer satisfaction and potentially leading to stronger customer relationships. Companies investing in user-friendly self-service tools signal that they value their customers' time and encourage them to take control of their experiences. However, this approach must be continually monitored and improved to remain effective. As customer needs evolve and products change, the information available within the self-service portal needs to remain up-to-date and relevant. Failure to do so could result in a negative customer experience, negating the benefits of the system.
In the dynamic world of SaaS support in 2024, the concept of "ticket deflection" has gained significant importance. A ticket deflection rate of 40% implies that a substantial portion of customer questions are resolved using self-service options, like online help centers, without ever needing a human support agent to intervene. This isn't just about reducing the workload for support staff – it's a key indicator of how well a company is empowering its customers. It essentially means that they've built systems that allow customers to find the answers they need on their own, reducing the reliance on human interaction for the simpler questions.
It's interesting to note that this can have a strong positive impact on costs. Research suggests that resolving an issue through self-service is often significantly cheaper, potentially up to 80% less, than handling the same question via a support agent. The logic is straightforward – if fewer people need to contact support, support costs naturally decline. However, this cost benefit has to be weighed against the investment it takes to build and maintain those self-service resources.
From a customer's perspective, it makes sense why ticket deflection is rising. Studies consistently show that a large number of users, often around 70%, prefer to find answers independently rather than contacting support directly. This aligns with the expectations of modern users who are used to fast, convenient access to information. Essentially, customers value the speed and convenience that comes with resolving their own issues. Companies who recognize this trend and invest in easy-to-use self-service systems are more likely to meet these expectations.
When we think about the impact on the support team, it becomes clear that a high deflection rate can help rebalance their workload. Support agents can focus on the more challenging and intricate issues that really require human expertise. This leads to a more fulfilling and less monotonous work environment for the agents, which in turn can improve job satisfaction and staff retention. It also makes the team more efficient and effective since they can spend more time on problems that need a skilled human to tackle.
Interestingly, there's a link between ticket deflection and customer satisfaction. Customers who can quickly solve their own issues are naturally more satisfied. They feel empowered and appreciate the convenience of a system that allows them to find information easily. Naturally, this contributes to a more positive brand perception over the long run.
Self-service portals are often built around knowledge bases and, as a result, depend heavily on how well that information is organized and presented. It seems that AI-powered recommendations within these knowledge bases are a game-changer when it comes to boosting the success of self-service, which in turn boosts the ticket deflection rate. In essence, the AI can improve the accuracy of suggestions, guiding users to the answers they need. It can do this in real-time as they type their search terms.
By examining which topics result in the highest deflection rates, companies can identify frequent customer issues. This insight is invaluable for understanding common pain points and can be used to guide product development and feature enhancements. In essence, this type of analysis allows companies to proactively improve their products or services in a way that can potentially prevent future support tickets.
One key trend is the increased reliance on automation within the support sphere. Chatbots and virtual assistants are becoming increasingly common in handling routine tasks, and this can significantly increase a company's ticket deflection rate. This evolution underscores a wider movement towards intelligent automation, a trend likely to persist in the coming years.
If a company wants to achieve a high deflection rate, it must design self-service resources with accessibility in mind. The easier it is for users to find information, the more likely they are to use these self-service tools. This often comes down to the overall design and how clear the information presented is. Poorly organized or confusing online resources can make it difficult for users to access the help they need, limiting the effectiveness of these systems.
There's a critical point to keep in mind: while a high deflection rate is a great indicator of efficiency, it can also mask underlying problems. If customers consistently utilize self-service because they can't find the answers they need through traditional support, it suggests there might be deeper issues within the service or product itself. It's essential to ensure that users always have access to human support, even if it's less frequently needed. This highlights the importance of a balanced support system, one that incorporates effective self-service while maintaining a high-quality human support component.
In conclusion, the concept of ticket deflection through self-service portals is central to the future of SaaS support. It offers the opportunity to enhance the customer experience, reduce costs, and empower users to help themselves. However, companies need to manage these strategies carefully. It's important to make sure that the focus on efficiency doesn't compromise the quality of human support and understanding when needed. This evolving space demands a mindful approach to ensure a seamless and effective customer experience.
7 Critical Metrics That Define SaaS Support Hours Effectiveness in 2024 - Agent Performance Score Card Reaching 90% With Data Analytics
Within the ever-evolving SaaS support landscape, data analytics is transforming how we measure and improve agent performance. The goal of achieving a 90% agent performance scorecard is gaining traction, and it's being fueled by the ability to analyze data in new ways. By using metrics like Average Handle Time, First Call Resolution, and Customer Satisfaction scores, companies can get a much clearer picture of how well their support agents are performing. These insights not only show where agents excel but also pinpoint areas where things could be improved. That kind of data-driven understanding is key for optimizing support workflows and making the overall support operation more efficient.
But, relying too heavily on data can sometimes overshadow the human aspect of support. It's easy to get caught up in chasing numbers and potentially overlook the importance of agents feeling valued and connected to the work they do. In a constant push for efficiency and high scores, it's vital that organizations ensure the drive for a higher agent performance scorecard doesn't negatively impact the quality of the support or the well-being of the agents themselves. Achieving that balance between effective data-driven insights and fostering a positive, human-centered support environment is a challenge companies are facing as they strive for optimal support operations.
Reaching a 90% Agent Performance Score Card by leveraging data analytics offers a compelling window into improving SaaS support. By continuously analyzing agent performance in real-time, support teams can swiftly recognize areas of strength and weakness, fostering a dynamic cycle of improvement. This kind of system doesn't rely on delayed feedback; it's incorporated into the day-to-day functioning of the team, constantly refining the approach.
Predictive analytics can be a real game-changer when incorporated into this scorecard system. By analyzing historical trends in ticket volume and types, support teams can anticipate potential issues and proactively adjust staffing and training. This foresight helps prevent bottlenecks and ensures a consistently high standard of support. It's a step away from just reacting to customer needs towards being better prepared for what might be coming.
There's a clear correlation between higher performance scores and increased employee engagement. When agents get real-time performance feedback and insights, they seem to feel more appreciated and valued. This heightened sense of worth leads to increased productivity and, intriguingly, decreased employee turnover. It suggests that a good scorecard system can have a positive impact on the well-being of the team in addition to improving the overall quality of customer support.
The Agent Performance Score Card system draws on the actual data from customer interactions to identify common trouble spots for customers. This helps refine support strategies and makes sure that agents are well-equipped to handle the most frequent issues they encounter. This type of analysis can improve the efficiency of the support team, getting customers to a resolution more quickly.
It's important to consider that a high performance score, while positive, might not always mean the highest quality of support. If the focus is simply on speed, there's a risk that the quality of the interaction will suffer. Superficial or rushed responses can erode customer satisfaction, even if the ticket is closed rapidly. Striking a balance between quick resolutions and comprehensive, high-quality interactions is key.
Organizations that prioritize data-driven training programs often see a significant boost in their Agent Performance Score Card. By targeting the training directly to the information revealed through the data, agents get the specific skills they need for the situations they regularly encounter. This targeted approach can lead to better results than more general training that might not address the core needs of the team.
Real-time feedback systems can elevate performance metrics. These systems allow agents to reflect on their interactions right after a customer issue is resolved. This type of instant analysis allows for quick skill adjustments and potentially reduces repeated errors. It's almost like a constant learning process for the agents embedded in their day-to-day workflows.
Reaching that 90% performance target often involves comparing performance to industry benchmarks. Companies that continually evaluate their metrics against their competitors can foster a culture of innovation, resulting in consistently better support delivery. It seems that setting a high bar and then striving to reach it can yield strong benefits.
The Agent Performance Score Card isn't just a siloed metric; it contributes to broader success indicators like customer satisfaction and average resolution times. A solid score in this area often signifies that the team is also succeeding in other vital areas of support. This wider view of the data offers a comprehensive picture of the overall effectiveness of the support function.
Interestingly, studies have shown a statistical relationship between better agent performance scores and improved employee satisfaction. As agents become more proficient and receive positive reinforcement from high scores, they tend to experience a greater sense of achievement and fulfillment. This contributes to a more positive work environment and ultimately can contribute to long-term employee retention. It shows that a good scorecard system isn't just about metrics; it can also be an integral part of improving the experience of working on the support team.
Automate Your RFP Response Process: Generate Winning Proposals in Minutes with AI-Powered Precision (Get started for free)
More Posts from rfpgenius.pro: