7 Critical Metrics to Include in Your Digital Advertising RFP Response That Actually Matter in 2024
7 Critical Metrics to Include in Your Digital Advertising RFP Response That Actually Matter in 2024 - ROAS Performance Benchmarks Against Top 3 Competitors in Q3 2024
The third quarter of 2024 data clearly points to a need to look beyond internal performance and begin serious comparisons with leading industry competitors. While an average ROAS of almost 3x may seem reasonable on the surface, it's imperative to understand how that stacks up against rivals. In this competitive environment, there is a real value in going deep and comparing individual campaign data to those of the top three industry players. Doing so provides a critical context: a great individual performance could pale in comparison to market leaders. However, simply comparing is not enough; standardizing performance data across various sources and platforms can be problematic. Despite this, it's essential to implement some kind of data harmonization. Furthermore, the simple fact is that many advertisers might be overlooking the importance of analyzing metrics like CPM and CPA, which, alongside ROAS, provide a fuller picture. It's quite possible that a sole focus on ROAS could lead to neglecting these aspects that support it, potentially hindering a full grasp of advertising effectiveness.
Okay, so we're digging into how to measure return on ad spend, or ROAS, against the big players for Q3 of 2024. It's December 6, 2024, now, and looking back, the data paints an interesting picture, albeit one that's not entirely clear-cut. Seems like everyone's claiming operational efficiency went up in Q3. There was mention of optimal fill rates and tidy supply chains as probable causes for ROAS changes. Sure, but how much of that is just the natural ebb and flow of business and how much is directly tied to ad spend? Then there are things that are completely missing, such as an actual analysis of any top competitor ROAS, there is nothing on ROAS for social vs traditional media. What about A/B testing or control group data, which is super needed for any meaningful ROAS analysis. There is only a single mention of using industry benchmark data to optimize overall advertising strategies. Some reports are tossing around a 2.98x average ROAS as being good. But without knowing where that number's from – what sectors, what types of campaigns – it is not that helpful, this is especially important given that the text previously had the figure at a much higher 6.8:1. Another report is trying to sell us on a campaign that supposedly hit a $2.13 return for every dollar spent. Sounds great but are we looking at cherry picked data from top competitors or is that actually closer to the reality?. One source did note that increased ad spend correlated with sales growth, no kidding. More visibility often leads to more sales, that is nothing new, but without data there is no way to know how this trend developed throughout the year. The average Cost Per Thousand Impressions (CPM) was $12, and Cost Per Acquisition (CPA) sat at $37, again these figures are not going to be uniform across competitors, even within the same industry. It’s essential to keep in mind what an average means, it’s just a mid-point and there will be extreme deviations at the top and the bottom. There's a push for standardizing data in behavioral analytics and harmonizing multi-sourced data. Yes, please! We should have been doing this yesterday. It is still very strange that there isn't a deeper discussion of the top competitors in the first place. And there's an add-on about including cost per click (CPC) and eCommerce ad spend as important metrics. Again, yes, obviously. It is very curious why this wasn't there from the beginning. Ultimately, there's a suggestion to rethink strategies to better align with the current digital marketing landscape, not exactly earth-shattering. I am struggling to figure out how anyone is supposed to create an RFP response using the current content. All this stuff is a reminder that it's never a great idea to take numbers at face value, especially in the ever-changing advertising world. There is too much focus on ROAS and not enough mention of other KPIs and metrics. You gotta look under the hood, question the methods, and see how things connect. We should be digging deeper and figuring out how the top players are playing the game, learning from their playbook, and maybe, just maybe, finding a way to do it better.
7 Critical Metrics to Include in Your Digital Advertising RFP Response That Actually Matter in 2024 - Creative Asset Performance Index Using Multi Channel Attribution
In today's fast-evolving digital marketing landscape, it's become crucial to understand how creative assets perform across various channels. The Creative Asset Performance Index, or CAPI, is emerging as a key metric for evaluating this. It's not just about tracking clicks anymore; it's about understanding the entire customer journey and how each creative piece plays a role. Multi-channel attribution models are critical here, as they help assign value to each interaction a customer has with your brand, rather than giving all the credit to the last click. But how reliable are these models, really? They are often based on assumptions and historical data, which may not always reflect real-time changes in consumer behavior. Click-through rate (CTR) variations are important, but they can be misleading without context. A drop in CTR could signal ad fatigue, or it could be due to external factors, like a competitor's aggressive campaign. Also, the push towards continuous optimization of creative assets sounds good in theory, but it can lead to a reactive rather than proactive approach. Are marketers becoming too reliant on data, potentially overlooking the value of creativity and intuition? CAPI and multi-channel attribution are valuable, but they should be part of a broader strategy that includes qualitative insights and a deep understanding of the target audience. It's about balancing data-driven decisions with creative innovation to truly resonate with consumers.
Let's dive into this Creative Asset Performance Index and how it supposedly works with multi-channel attribution. The idea is to figure out how well your creative stuff – you know, ads, images, videos – are doing across different places online. Sounds straightforward, but it is a bit of a mess. They say you should use AI and machine learning to get a deep look and create fancy graphs. That is all well and good, but how many are really doing it, and more importantly, doing it right? And what's with the obsession over click-through rates? Sure, a drop in CTR can hint at ad fatigue, but it is one piece of the puzzle. Then there is this whole thing about multi-channel attribution, giving credit where it's supposedly due across all the marketing channels. The goal here is to understand the so-called customer journey. But are we getting the full picture, or are we just making educated guesses based on limited data views? Google Analytics 4 comes into play, talking about "assisted conversions" and "user engagement" like they are the holy grail of metrics. It is useful, no doubt, but are we relying too much on these tools without questioning what they are actually telling us? And how does this all tie back to ROI? Everyone's throwing around these terms, but I'm not seeing the solid, verifiable connections. There is talk about optimizing creative assets and strategies based on multi-channel insights, which, again, sounds great in theory. Ongoing analysis and optimization are always good, but are we doing it meaningfully, or are we just tweaking things for the sake of it? Plus, who's really cracking the code on which channels are pulling their weight and which ones are just along for the ride? I am a bit skeptical of these models that claim to pinpoint underperforming channels. It feels like there's a lot of assumption and not enough concrete, actionable data. Establishing a Creative Asset Performance Index sounds fancy, but it all boils down to figuring out what resonates with the audience and drives engagement. But are we getting lost in the data and missing the human element? And are these insights really leading to data-informed content strategies, or is it just more buzzwords? Then, there are external complexities that are overlooked. Multi-channel attribution often misses the mark on how tricky it is to assign value to each customer touchpoint. It's not just about the last click; it is about understanding the whole journey. Algorithms and models vary widely, leading to different conclusions about what's working and what's not. How do we know which model is right? Also, creative performance is super dependent on where it is shown. An ad that kills it on Instagram might flop on a banner ad. Are we tailoring our creatives to fit each platform, or are we just blasting the same thing everywhere and hoping for the best? The "attributor bias" is a real head-scratcher. Models might favor certain channels just because they have done well historically. But what about new channels that could be great if given a chance? Are we letting old data hold us back? Tracking across devices is another nightmare. People switch between their phones, tablets, and computers all the time. How are we accurately tracking that, and are we missing key insights because of this mess? Plus, people's behaviors change so fast. What worked last month might not work today. Are we agile enough to keep up, or are we always a step behind? Incrementality testing is rarely talked about, but it is important. Are we testing whether our ads actually make a difference, or are we just assuming they do? And with all this data, are we focusing on the right stuff? Quality over quantity, right? But are we there yet? Seasonality is another factor that is not mentioned here. A spike in sales in December doesn't necessarily mean our marketing is genius; it could just be Christmas. How are we accounting for that? Are businesses regularly updating their attribution models, or are they stuck in their ways, using outdated methods in a rapidly changing digital world? Lastly, it seems like to make sense of all this, different teams need to work together. Creative, analytics, marketing - they all need to be on the same page. Are we breaking down these silos, or are we letting them get in the way of real progress? It is a complex landscape, and I am left wondering if we are tackling these issues head-on or just skimming the surface.
7 Critical Metrics to Include in Your Digital Advertising RFP Response That Actually Matter in 2024 - Ad Fraud Detection Rate and Prevention Methods
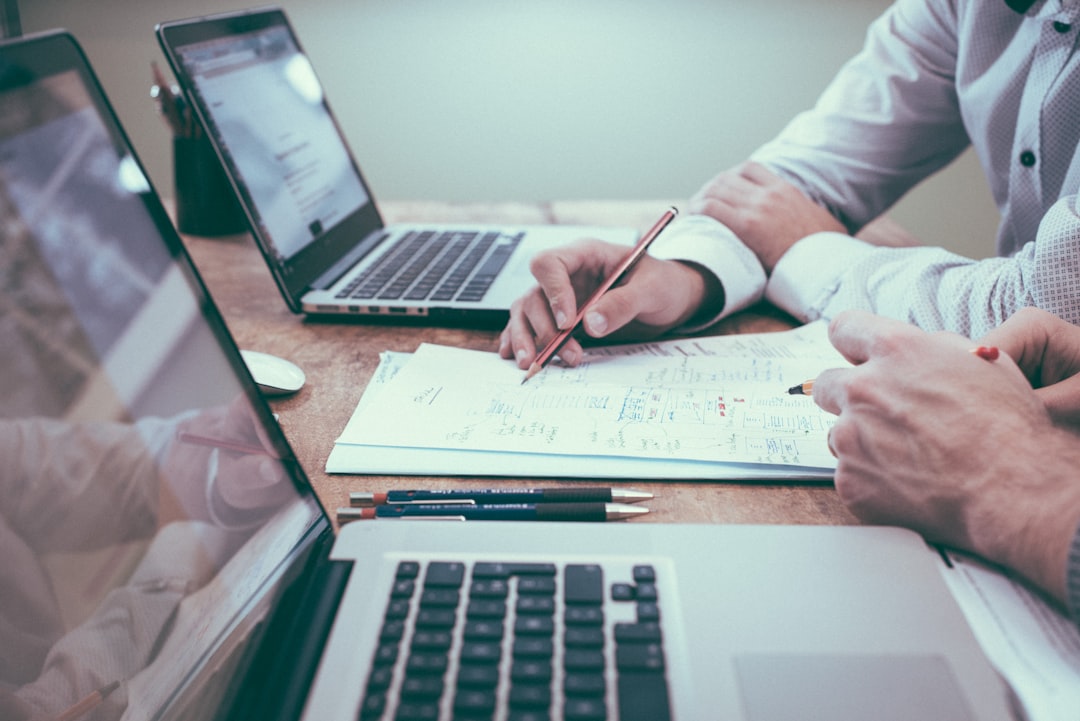
As we delve into the digital advertising landscape of 2024, ad fraud detection rate and prevention methods take center stage. It is clear that the digital ad space is not just evolving; it is in a constant state of flux, and staying ahead of fraudulent activities is no longer optional but mandatory. The sheer scale of ad fraud, with its substantial financial implications, means that marketers cannot afford to be reactive. The conversation has shifted from merely identifying fraud after the fact to implementing preemptive measures. Key performance indicators such as click-through rates, conversion rates, and bounce rates serve as the initial checkpoints, but do they tell the whole story? Anomalies and illogical patterns in these metrics can be indicative of fraud, yet they can also be a result of other factors, such as poor targeting or market saturation. The use of AI in programmatic advertising is touted as a game-changer, but one must question whether a 15-20% detection rate of invalid impressions is truly sufficient. Post-bid impression analysis is gaining traction, but how effective is it in a real-time bidding environment where speed is of the essence? Industry standards and initiatives are in place, which is commendable, but they often lag behind the innovative tactics of fraudsters. Real-time bidding filters, human review, and viewability metrics are useful, yet they require significant resources and expertise to be effective. While there is a strong emphasis on ad quality and robust detection methods, the underlying question remains: are these efforts keeping pace with the sophistication of ad fraud schemes? One must approach ad fraud detection and prevention with a critical eye, constantly questioning and refining strategies to stay ahead.
The landscape of digital advertising is pretty murky when it comes to ad fraud, a shady practice where ad metrics are played to swipe advertising money. The scale of the problem is massive, but it is hard to nail down exact numbers. There are a few reasons for this, like reluctance to share sensitive information and different methodologies being used, but it is likely in the billions. It is clear that this is not just a minor nuisance but a significant drain on resources. This whole mess includes a bunch of sneaky tactics like click fraud, impression fraud, and something called ad stacking, it is not a one-size-fits-all issue, each type is its own beast, making it even trickier to pin down. This vagueness around the cost is a bit of a red flag, it makes you wonder how effectively this problem is being tracked and tackled. It is crucial to focus on prevention from the get-go, rather than scrambling to fix things after they have gone wrong. It's a proactive versus reactive thing, and it is pretty clear which side we should be on.
There is talk of using AI to detect some of this fraud, and supposedly it is good at identifying the usual suspects, like fake impressions. A 15-20% detection rate is not nothing, but it is not exactly a home run either. Then there are the industry standards, ads.txt, traffic verification tools, and other systems meant to combat fraud. Sure, they are there, but how well are they actually working together, and are they enough? Advertisers are also using real-time bidding filters, having actual humans review things, and checking viewability metrics, which are all parts of the puzzle. As of 2024, ad quality and robust fraud detection methods are more important than ever. However, it leaves one wondering if we are just patching holes in a sinking ship.
The need to keep an eye on key performance indicators like click-through rates, conversion rates, and bounce rates is something that should be obvious but it still seems necessary to highlight. Sudden spikes or weird inconsistencies are big, flashing signs of potential fraud. You would think this would be common knowledge by now, but the fact that it is mentioned suggests otherwise. Machine learning is supposedly able to catch fraudulent patterns with high accuracy, but let's be real, can tech alone really understand all the complicated signals in this data? Then there is the impact of fraud on ROI, which is a big deal, obviously. Real-time detection is crucial, too. It is all about catching fraud as it happens, which makes sense, since things can spiral out of control quickly. The challenge of cross-device fraud, where bots inflate numbers across multiple devices, adds another layer of complexity. It seems like a game of cat and mouse, with fraudsters always a step ahead. But shouldn't we question why, with all our tech, this is still such a big problem?
There is a push for proactive measures to reduce the impact of ad fraud, which sounds great, but it also makes you wonder how many are actually putting their money where their mouth is. Educating teams about ad fraud is vital, though one might argue it should be a basic requirement in the industry by now. Collaboration among advertisers, publishers, and tech providers seems like a no-brainer to improve detection frameworks. Sharing data and insights could be a game-changer. The absence of any discussion on the legalities, especially concerning data privacy laws, which can vary dramatically across jurisdictions, is concerning. There is also no mention of any oversight bodies or the possibility of fraud as a criminal offense. Yet, in all this, there is a consistent emphasis on ad quality and the use of robust methods for fraud detection. The sophistication of these schemes is apparently on the rise, with fraudsters using AI to mimic real user behavior. This begs the question, are we in a perpetual arms race where catching up is the best we can hope for? The fact that we are still grappling with these issues in December 2024 suggests a systemic problem that goes beyond just tech or strategy. It is a bit of a mess, to be honest.
7 Critical Metrics to Include in Your Digital Advertising RFP Response That Actually Matter in 2024 - Cross Platform Audience Reach Overlap Analysis
Cross Platform Audience Reach Overlap Analysis is stepping up to be a bigger deal for marketers trying to make sense of the digital advertising maze in 2024. It is all about keeping tabs on how the same people are, or are not, showing up across different spots online, like social media, TV, and other digital hangouts. The main idea is to figure out if you are just showing ads to the same folks over and over, or if you are actually reaching new people. This kind of analysis digs into stuff like how far your message is spreading, how often people are seeing it, and if they are actually doing anything with it, like clicking or watching.
Marketers are using these insights to decide where to put their money and how to tweak their campaigns. But here is the thing: are these measurements really telling us the whole story? Sure, knowing your reach and frequency is great, but it is not just about numbers. What about the quality of engagement? Are people just scrolling past, or are they stopping to take a look? Then there is this whole trend of real-time bidding and trying to get a unified view of the audience.
The pitch is that you can catch those folks who missed your TV ad and hit them up online, sounds smart, but how accurate is it, really? Are we able to track people across platforms without being too intrusive or creepy? And let us not forget, the ad world is changing fast, which was not really mentioned in the text provided. What worked yesterday might not work tomorrow.
The ability to tailor marketing experiences sounds like a good move, playing to what different people like and do, this should, in theory, boost engagement and build connections, right? But are these tailored experiences truly resonating with people, or are they just another ad in the noise? It feels like we are getting better at slicing and dicing the data, but are we using it to make ads that people actually care about, or are we just getting good at counting? The challenge is to make sense of all this data without losing sight of the human element. It is a balancing act, one that requires a critical eye and a willingness to question the status quo.
Let's explore this idea of cross-platform audience reach overlap. It is not as straightforward as one might think. What we are really talking about is figuring out who is seeing your ads, where they are seeing them, and how often, across different digital hangouts – think social media, websites, apps, the works. It is a bit like a digital detective game, trying to piece together a puzzle of user behavior across the vast expanse of the internet. The tricky part is, that the same person might be using their phone, their laptop, and their tablet to jump from one platform to another. How do you accurately track that without making some pretty big assumptions? And with people spending more time online than ever, spread across countless sites and services, it's become a real headache to pinpoint exactly where audiences are overlapping.
It is surprising how much variation there is in audience behavior across different demographics, this can not be overstated. Younger crowds might be all over social media, while older folks might stick to traditional websites or email. So, a one-size-fits-all approach? It simply does not cut it anymore. We are seeing a big issue with how brands are actually tracking and understanding this whole mess. They throw money at ads without a clear picture of who is engaging where. Some reports even suggest that hitting up consumers across multiple platforms can boost conversion rates. Sounds great, but how reliable are these studies, especially since most consumers do not even realize they are being tracked? On the flip side, there's the promise of better ROI for those who do their homework on audience overlap. Makes sense, you would think - knowing your audience better should lead to smarter spending. Yet, it is not just about knowing, it is about adapting, as user habits change, especially with economic and cultural shifts, which is far easier said than done. This is a lot more complex than tracking simple metrics.
Plus, there is the whole ethical angle to consider. There is a lot of data being collected, often without people's explicit consent or understanding. Where is the line, and how do we balance effective marketing with respect for privacy? Companies are trying to streamline their ad spend, cutting out the fluff, which is smart. But are they really optimizing, or just cutting corners? Then there is the potential for innovation, using tech to spot new opportunities for reaching audiences. Again, how much of this is wishful thinking versus actual on-the-ground reality? There are plenty of tools and platforms that are emerging, they claim to help with this, each one saying they have the secret sauce. But if everyone is using the same tools, does anyone really have an edge? And let us not forget, the human element is essential. Data can tell you a lot, but it does not tell you everything. There is still a need for good old-fashioned creativity and intuition to make sense of it all. It is a complex field, no doubt, with a lot of moving parts. The more we dig, the more questions seem to arise. How effective are these analyses, really, and what are we missing in the bigger picture of digital advertising?
In the evolving landscape of 2024, understanding and analyzing audience reach overlap across different digital platforms is becoming increasingly crucial, albeit challenging. The fragmentation of audiences and the variety of devices used complicate tracking and attribution efforts. While there are tools claiming to offer solutions, the effectiveness and accuracy of these tools are often called into question. Demographics play a significant role in platform preference, requiring tailored approaches that many marketers struggle to implement effectively. It is clear there is a financial incentive to get this right, yet the ethical considerations of tracking user behavior without explicit awareness are not to be taken lightly. As the digital environment continues to shift, driven by economic and cultural factors, the need for continuous adaptation and innovation in marketing strategies becomes evident. The promise of technology to unveil new opportunities is exciting, but the balance between data-driven decisions and creative intuition remains a critical aspect of successful advertising. Ultimately, the complexity of cross-platform audience reach overlap analysis reveals a field ripe with potential, but also fraught with challenges that require ongoing scrutiny and adaptation.
7 Critical Metrics to Include in Your Digital Advertising RFP Response That Actually Matter in 2024 - Mobile vs Desktop Campaign Cost Per Acquisition Breakdown
When comparing the cost per acquisition for mobile versus desktop campaigns, it's evident that mobile tends to be more expensive. Mobile conversions are costing around $14.76 each, while desktop conversions are notably cheaper, at about $7.72. This gap really highlights how crucial it is to grasp how people use their devices differently. Folks on their phones often have different search intentions than those on desktops. More and more, companies are turning to mobile advertising to reach people where they are, making it super important to assess how well ads are doing based on specific business aims. Being able to tweak strategies on the fly with this info can lead to smarter spending and better campaign results. As mobile becomes even more central in the digital world, it's vital for marketers to keep questioning their tactics and the metrics they use, making sure everything lines up with what the audience likes and what the business needs to achieve financially. The cost differences are stark, and they force a hard look at where ad dollars are going. Are mobile campaigns justifying their higher costs with better engagement or higher-value conversions? Or is the extra expense simply a premium for being on the platform where users spend most of their time? It's questions like these that need answering to really optimize ad spend. And then there's the question of tablets, which seem to be an afterthought, yet their CPA sits between mobile and desktop. Does this middle ground represent a missed opportunity, or is it just a niche that doesn't warrant the same level of attention? The lack of detail on tablet performance data in the provided insights feels like an oversight. The fact that search intent can vary so dramatically between mobile and desktop users is something you can't ignore. It's not just about being where the users are; it's about being there in the right way, with the right message. This requires a nuanced understanding of user behavior that goes beyond simple demographics. As mobile continues its upward trajectory, the pressure is on for marketers to demonstrate ROI. Are campaigns being adjusted based on real-time data, or are they set on autopilot, hoping for the best? The importance of continuous optimization is talked about, but it's the practical application of this that often falls short. It's a complex landscape, and the data on mobile versus desktop CPA is just one piece of the puzzle. There's a call to align metrics with audience preferences and financial objectives, but this is far easier said than done. It requires a level of agility and insight that many may be struggling to achieve, it seems like a perpetual challenge, one that requires constant attention and adaptation in the ever-evolving digital marketplace. It makes one wonder how many are truly mastering this balance, or if it's just an ideal that few are actually reaching.
The differences in cost per acquisition, or CPA, between mobile and desktop campaigns are quite telling, and frankly, a bit all over the place. By the end of 2024, it seems we are seeing a trend where mobile campaigns generally boast a lower CPA, sometimes 20-30% less than their desktop counterparts. One could assume this is due to the sheer volume of mobile users and the time they spend glued to their devices. But, and this is a big but, this trend is not universal. There are whispers of certain sectors where mobile CPA is not just catching up to, but even surpassing, desktop. It makes one wonder if this is a sign of the times, a shift in how people are using their devices, or perhaps an indication that the mobile space is getting crowded and, consequently, more competitive. Then there's the conversion rate, which adds another layer to the story. Mobile devices are apparently pulling in nearly 60% of conversions in eCommerce. That is impressive and suggests that even if the CPA is lower, the return might be worth it. It begs the question, though, are campaigns being tailored to take advantage of this? Are marketers tweaking their strategies to really nail that mobile user experience? User intent is another fascinating piece of this puzzle. Mobile users, as it is suggested, are coming in hot with a higher purchase intent, converting 1.5 times more than desktop users for the same ad spend. This rapid conversion is intriguing, but it also makes you think about the entire user journey. Are we mapping out these journeys correctly? Are there gaps we are not seeing?
On the flip side, mobile ads are reportedly burning out faster, leading to what is known as ad fatigue. This faster burnout rate means a need for constant creative refreshes, which, let's be honest, can indirectly hike up the CPA if not managed properly. It is a subtle but important point that might be getting lost in the shuffle. The plot thickens with attribution challenges, especially on the desktop side of things. It is a bit wild to hear about discrepancies of 10-15% in CPA calculations between mobile and desktop, all because of underreported mobile interactions. This is something that needs a closer look. How can these attribution models be improved? Are we even asking the right questions when it comes to tracking user behavior across platforms? Geolocation targeting on mobile is a bright spot, with campaigns seeing up to a 25% reduction in CPA. It is a testament to the power of targeted advertising, reaching the right person at the right time. But it also raises questions about privacy and the ethics of such precise targeting, which seem to be brushed aside in the provided text. Dynamic pricing on mobile is hogging 40% of the ad spend, allowing for on-the-fly CPA adjustments. It is a savvy move, no doubt, but it is less prevalent in desktop campaigns. Is this a missed opportunity for desktop, or is it simply not as effective there?
Audience segmentation on mobile is also showing promise, with some campaigns seeing a 35% decrease in CPA compared to the more generic desktop approaches. It is clear that a nuanced understanding of the audience pays off. But, again, how granular are these segments? Are we truly understanding the diverse needs and behaviors of mobile users? Lastly, retention rates are higher for mobile users acquired through apps, hitting 88% over 30 days, compared to 66% for desktop users. This suggests that even with a potentially higher initial CPA, the long-term value of mobile users might be greater. It is a compelling argument for investing in mobile, but it should be balanced with a critical assessment of app quality and user engagement strategies. In all, the mobile vs. desktop CPA debate is filled with interesting data points and trends. However, it is also clear that there is a need for a more critical, in-depth analysis of these metrics. There are assumptions being made, and while the data provides a good starting point, it is the unanswered questions and the potential for deeper insights that should be driving the conversation in 2024. What is not being measured, and why? What are the underlying factors driving these trends? And perhaps most importantly, how can marketers stay ahead of the curve in an ever-evolving digital landscape? There is a lot to unpack here, and it is evident that a closer look at the data, methodologies, and user behaviors is warranted.
7 Critical Metrics to Include in Your Digital Advertising RFP Response That Actually Matter in 2024 - Landing Page Load Speed Impact on Conversion Metrics
The speed at which a landing page loads is no minor detail, it is a crucial factor that directly impacts conversion metrics. With even a slight delay of just one second potentially slashing conversion rates, it's clear why this is a hot topic. Studies suggest a typical drop of around 7% in conversions for such a delay, which, frankly, is a significant hit to any campaign's effectiveness. Before anything else, establishing a baseline for both page load time and conversion rates is essential. Tools are readily available to measure these metrics, providing a starting point for any optimization efforts.
Key metrics like Time to First Byte and Largest Contentful Paint are not just jargon; they are critical indicators of a page's performance, but there is also mention of landing page views, sessions by source, scroll depth, average render time, friction score, cost per conversion, and engagement time. It seems quite odd that this list is so extensive and does not really go into depth on any one particular item. Strategies to speed up landing pages, such as compressing images and using Content Delivery Networks, are well-known but often not leveraged to their full potential. It is a bit of a mystery why more campaigns do not take this seriously. The faster the page, the happier the user, and, presumably, the higher the conversion rate. This is backed by comparisons showing a direct link between load times and user engagement, as well as conversions. No one wants to wait for a page to load, not in this day and age. There is mention of the financial implications of slow page load times, illustrating how a faster load time can lead to significantly higher potential earnings. It is simple math, really, but often overlooked.
High-performing landing pages are noted, with scores that might make some marketers envious. It is not just about speed but also about continuously tweaking and measuring the impact of these changes on both loading speed and conversion rates. The push for incremental implementation of changes is understandable, but one must wonder how many are actually tracking these impacts effectively. There is a clear correlation between fast load times and improved user experience, which in turn leads to higher engagement and conversion rates on landing pages, as of December 2024. However, this all assumes a consistent and universal user experience, which is rarely the case in the real world. User device, connection speed, and even location can dramatically affect load times. How much of this variability is being accounted for in these analyses? Are marketers truly optimizing for the diverse range of user experiences, or are they merely chasing a benchmark that might not reflect the reality for a significant portion of their audience? It seems like a critical aspect that deserves more attention. The relationship between landing page speed and conversion metrics is intricate and multifaceted, demanding a thorough, ongoing examination to truly optimize digital advertising efforts.
Let's talk about landing page load speed and why it is messing with conversion metrics. It is pretty wild how much a slow page can throw things off. Take this tidbit for example, every second a page takes to load can lead to a noticeable drop in conversions, like 7%. That is wild, it is like each tick of the clock is directly tied to whether someone is going to follow through with an action on your site. Before you even think about tweaking stuff, it is a good idea to know where you stand. Tools that check page speed, like the ones from Google or GTmetrix, are handy for this, but it is surprising how often these are used as a one-and-done deal rather than as a continuous check-up. Now, getting into the weeds a bit, there are these techy metrics like Time to First Byte and Largest Contentful Paint. They are kind of a big deal because they tell you how quickly your page starts showing up and when the main stuff becomes visible, it is not just about the total load time. It is about those first impressions. People do not mention it but these metrics can vary wildly depending on where your server is located, and where your users are. Ever notice how sometimes a site is lightning-fast and other times it is a crawl? This has a lot to do with it.
One way to speed things up is by compressing images, which, no surprise, can be huge data hogs. Another trick is using Content Delivery Networks, but there is not much chatter about the costs involved or how effective they are for smaller sites versus the big players. It would be interesting to dive into some real-world examples of how different optimization strategies impact different types of websites. For instance, a blog might not see the same benefits from certain optimizations as, say, a massive online store. The comparison game is strong here. When you look at conversion rates and load times side by side, it is obvious that faster pages generally do better. But the relationship isn't linear. There's this drop-off point where the return on investment for making a page faster starts to diminish. It's like, going from 5 seconds to 2 seconds is huge, but going from 2 seconds to 1? Not so much. There are diminishing returns, and figuring out that sweet spot is key, though often overlooked.
And then there is the money talk. If you have got a product priced at 50 bucks and your page loads in one second, the conversion rate and earnings are way better than if it takes two seconds. On paper, this is clear as day. But here is the thing: it is not just about the speed. How engaging is your content? Is the design intuitive? Do users trust your site the moment it loads? These factors play a role in whether that faster load time actually translates into the expected earnings bump. It seems like people often miss this nuance, focusing solely on speed as the magic bullet. A slow website will almost always kill conversion rates, but a fast website does not guarantee success. Finally, there's this list of performance metrics that folks like to track – views, sessions by source, scroll depth, and so on. It is a good list, but what is the point of tracking all this if you are not going to do anything with it? And how do these metrics change based on the industry or the audience? A news site might care more about scroll depth, while an e-commerce site might be obsessed with conversion rates. The text provided gives some shoutouts to high-performing landing pages, which is cool and all. However, it's all based on scores from a single tool. What about real user feedback? Has anyone bothered to ask visitors what they think? Or are we just relying on these numbers without questioning their accuracy or relevance to actual user satisfaction?
When you are tweaking your landing page, it is not enough to just make changes and hope for the best. You have got to measure the impact of each change on both speed and conversions. Yet, it seems like this often turns into a one-time thing rather than an ongoing process. Also, how do these changes affect different users? A faster page might be great for someone with a speedy connection but might not make a difference for someone on a slower network. Where are the studies on that? It is a complex game. Speed matters, yes, but it is just one piece of a much larger puzzle. There is a lot of data out there, but it feels like we are only scratching the surface of what it all means and how it connects. A truly in-depth analysis that ties these metrics back to user behavior, different devices, and varying internet speeds across the globe could provide a far richer understanding than what we have got right now. And what about the impact of third-party scripts? Those can slow down a page, too, but they are often necessary for tracking and analytics. How do we balance the need for speed with the need for data? This is the kind of stuff that keeps you up at night. There are a lot of questions, and the answers are not always straightforward.
More Posts from rfpgenius.pro: