7 Key Metrics to Include in Your Software Service Level Agreement for 2025
7 Key Metrics to Include in Your Software Service Level Agreement for 2025 - Uptime percentage and service availability tracking
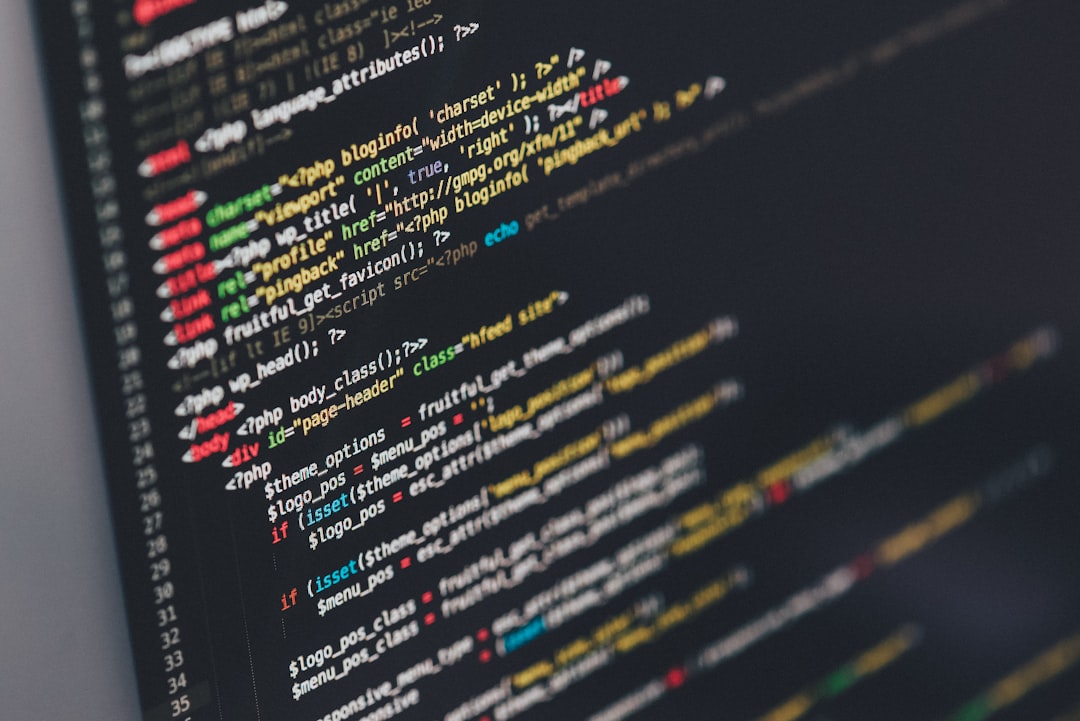
Tracking uptime percentage and service availability is fundamental to any software service level agreement. These metrics, typically expressed as a percentage reflecting the operational time of a service, are key indicators of reliability. A stated goal like 99.5% uptime might seem impressive, but it can still represent a substantial amount of downtime, potentially affecting service users. By closely monitoring these metrics, both parties—the service provider and the user—gain a clear understanding of the service's performance. This clarity promotes transparency and accountability, ensuring the service provider lives up to their commitments. As the software landscape continues to evolve and users become more demanding, strict adherence to agreed-upon uptime and availability targets will be increasingly crucial for a positive user experience. The consequences of falling short can be substantial, impacting trust and potentially damaging long-term relationships.
When discussing service reliability, uptime percentage is often simplified, but it's really a spectrum. A 99.9% uptime target, for example, translates to nearly 9 hours of potential downtime annually—a substantial amount that can significantly impact business. While many service level agreements (SLAs) aim for a baseline of 99.5% uptime, achieving higher uptime, like "five nines" (99.999%), can be very expensive due to the infrastructure required for such reliability.
It's also important to realize that service availability isn't just about servers being online. It involves a broader picture, including how quickly a service responds to requests and whether those transactions are successful. This gives a more complete view of how effectively a service actually functions for end-users. That "five nines" target, allowing only about 5 minutes of downtime per year, is considered a gold standard in industries like finance and healthcare, though it's a very demanding goal, rarely achieved in practice.
While technology can be advanced, a large portion of service outages, over half, are surprisingly due to human mistakes. This highlights how even with sophisticated systems, the human factor remains a primary source of potential disruption. The relationship between uptime and user satisfaction isn't perfectly linear, though. While most users expect a minimum of 99% availability, research shows that user satisfaction plunges when downtime surpasses 1%.
Many organizations could benefit from adopting proactive real-time monitoring that can flag problems before they lead to disruptions. Instead, they often fall back on post-mortem analysis after an outage, delaying timely resolutions and impacting long-term service trends. Additionally, not all downtime is equal. Planned maintenance outages are often excluded from uptime calculations, emphasizing the need for clear SLA definitions to ensure true understanding of actual service availability.
The financial impact of downtime can vary dramatically across different industries. E-commerce, for example, can face average losses of $5,600 per minute of downtime, whereas telecommunications sectors can endure losses exceeding $2 million per hour. While a 99.9% uptime target is presented as a common ideal, the average across different industries actually sits closer to 99.5%, showing that there's often a disparity between desired uptime and what's achieved in practice.
7 Key Metrics to Include in Your Software Service Level Agreement for 2025 - Average response time for critical incidents
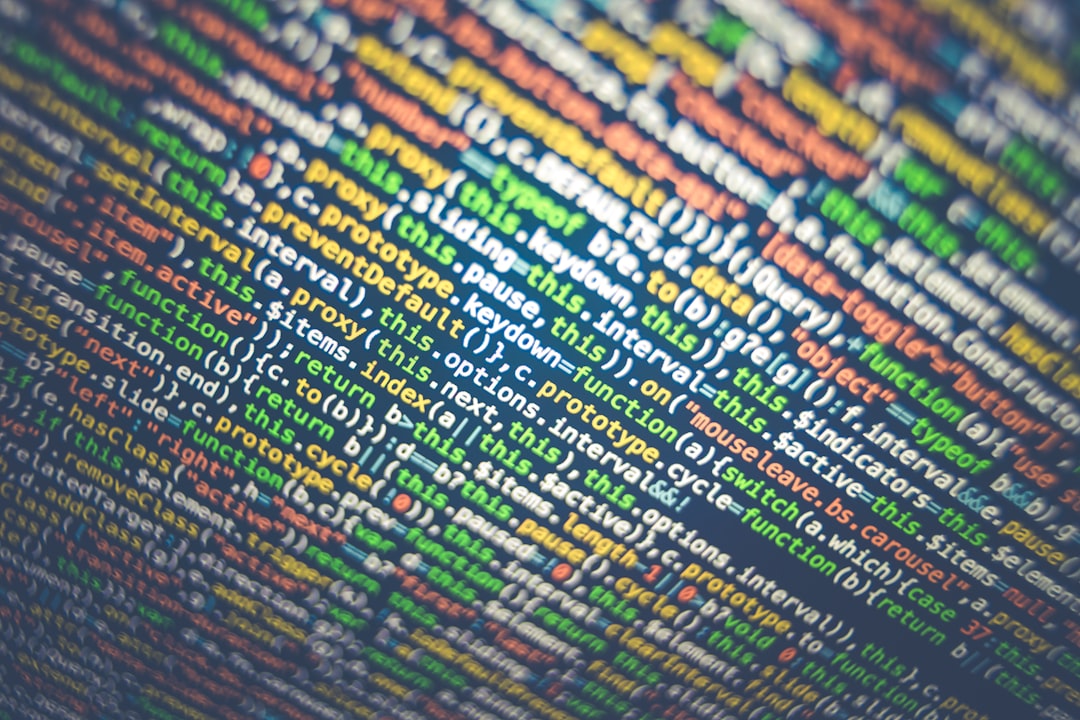
The average time it takes to respond to critical incidents is a crucial metric for gauging how well an organization can handle serious problems that might interrupt its operations. This metric measures the time from when a critical incident is reported to when it's addressed or resolved, offering a glimpse into potential bottlenecks within the system. By tracking response times, organizations can spot issues in their communication flows and staffing levels, allowing them to make adjustments to improve incident management. This metric, especially within the framework of a Service Level Agreement (SLA), becomes a way to measure not just operational efficiency, but also a way to ensure services meet agreed upon expectations. Given the continuous changes in the world of software, the importance of understanding and improving this average response time will only increase in the future for maintaining system stability and keeping customers happy. While SLAs can establish targets, it is the consistent monitoring of the actual average response times that is critical for proving or disproving their effectiveness in practice.
1. The average time it takes to respond to critical incidents can vary wildly depending on how severe the incident is deemed. A really serious issue might need a fix within 15 minutes, whereas a minor one could have a response window of up to a full day. This shows how important it is to properly categorize incidents.
2. Different industries have their own unique expectations when it comes to how quickly a problem needs to be dealt with. For example, industries like finance and healthcare usually need things fixed within minutes, whereas IT or gaming might be okay with longer resolution times, depending on the nature of the services they provide.
3. Having clear metrics in Service Level Agreements (SLAs) not only defines how fast a response should be but also can directly affect how well things are handled. Organizations that stick to strict SLAs often see their average response times improve significantly compared to those that don't have formal agreements in place.
4. User research is interesting because it reveals that while many companies aim for a 30-minute response to a critical issue, users often expect a response in 10 minutes or less. This difference can lead to dissatisfaction even if the response is within the SLA.
5. The time it takes to escalate a critical incident can create delays if the first person dealing with it doesn't have enough training or resources. In some cases, getting a more experienced team involved can take an extra 20-30 minutes, impacting the overall time to resolution.
6. Using AI-powered tools to track and handle incidents can significantly reduce the average response times. Companies that utilize automation often report a 30% faster response compared to traditional manual methods.
7. It's intriguing that many organizations experience a boost in efficiency *after* a critical incident. A detailed post-incident analysis often results in identifying ways to improve processes, enhancing future response times. It shows how incidents can lead to operational refinements.
8. The effectiveness of handling incidents isn't just about technical skills. Research suggests that clear and efficient communication can reduce the total resolution time by up to 30%, highlighting the importance of the human element in incident management.
9. There's this interesting phenomenon called the "cumulative downtime effect", where numerous small incidents can put a strain on response teams, making it harder to handle critical issues quickly. This results in longer average response times due to team exhaustion and resource juggling.
10. The perceived response time to a critical incident can worsen the impact. Even if the response is within the SLA, a delay in communicating about the issue can lead to increased anxiety and dissatisfaction among users. This suggests that managing expectations is just as critical as a quick response.
7 Key Metrics to Include in Your Software Service Level Agreement for 2025 - Mean time to repair (MTTR) for system failures
Mean time to repair (MTTR) is a crucial metric for evaluating how effectively a system is restored after a failure. It covers the entire process from the initial failure detection to the point where the system is fully functional again, encompassing the time spent diagnosing and testing the fix. MTTR provides valuable insights into how efficient an organization's maintenance and repair procedures are. It's a key metric for assessing both IT operations and the effectiveness of DevOps processes, particularly when looking at incident response and recovery times. By keeping a close eye on MTTR, organizations can pinpoint weaknesses in their repair processes and implement changes to improve incident handling. This helps minimize the effects of outages and ensures business operations can continue without major disruptions. Looking ahead to 2025, incorporating MTTR into software service level agreements (SLAs) becomes crucial. This ensures both the service provider and the user have a clear understanding of what constitutes acceptable recovery time, fostering accountability and realistic expectations for service uptime and issue resolution. While simply having a low MTTR is a positive sign, its true value lies in the ability to use the data to actually improve the processes of fixing and restoring service. A high MTTR can be a red flag, indicating possible weaknesses in a service provider's ability to respond promptly and effectively to service failures.
Mean Time To Repair (MTTR) isn't just about the time it takes to fix a system after it breaks down. It's about minimizing downtime and getting things back online quickly, making operations smoother.
It seems that having a dedicated team specifically for handling incidents can make a big difference in repair times. Research suggests that organizations with these focused teams can cut their average MTTR by 20-30% compared to those who rely on general IT staff, hinting that specialized expertise matters a lot.
Interestingly, the more often a system has issues, the faster it might get fixed on average. It seems that organizations dealing with regular outages develop faster repair procedures and improve communication, resulting in quicker resolutions over time.
Looking at different industries reveals a huge difference in expectations about how long a repair should take. While some environments that need constant uptime aim for under two hours of MTTR, others like manufacturing may be okay with a system being out of commission for up to 72 hours.
There are tools that can help find the root cause of a problem much more quickly, which directly leads to a lower MTTR. Companies using predictive maintenance see a huge drop, about 40%, in their MTTR compared to traditional approaches where they just react after something breaks.
The cost of downtime due to a longer MTTR can be very high. Companies can save a lot of money for every little improvement in their average MTTR, especially in areas like e-commerce and finance where time is directly connected to revenue loss.
The concept of "mean time to acknowledge" (MTTA) is important here. If an incident is acknowledged quickly, it often helps speed up the repair process. It appears the speed of the initial response really matters for how quickly a system can be restored.
It's worth thinking about the "cost of downtime" in relation to MTTR. Some studies show that a single hour of downtime can easily cost a company over $300,000, making the goal of lowering MTTR not just about efficiency but also about financial survival.
It's surprising that a relatively small percentage of organizations, less than 30%, actually measure or track their MTTR. This creates a blind spot in understanding how well their incident response teams are performing and makes it harder to improve system reliability.
The impact of a long MTTR isn't just about systems. It can also affect the people fixing them. If the repair times are consistently high, it can affect team morale and even lead to burnout for engineers who are constantly dealing with failures. This highlights the need for good resource management and support systems in environments with a lot of pressure.
7 Key Metrics to Include in Your Software Service Level Agreement for 2025 - Data security breach notification timeline
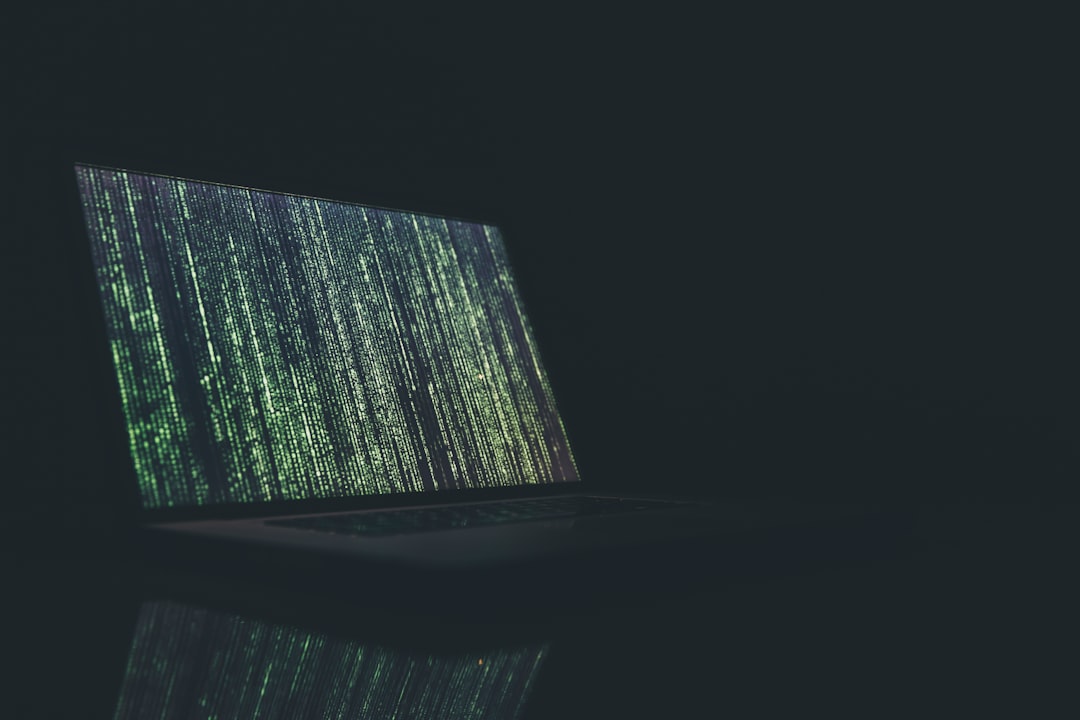
In a software service level agreement (SLA), a defined timeline for notifying relevant parties following a data security breach is essential. The speed and clarity of communication after a breach can significantly impact its consequences, including regulatory fines and reputational damage. This timeline, part of the SLA, should spell out precisely when and how affected customers, regulatory bodies, and internal stakeholders are notified. A well-defined process reduces confusion and demonstrates a commitment to transparency.
Keeping this notification timeline current is vital, as both regulations and best practices around data security evolve rapidly. Moving towards 2025, the necessity of prompt and thorough communication following a data security breach is unlikely to decrease. The growing focus on data privacy across industries makes swift and well-defined breach notification procedures more crucial than ever. While it's understandable to want to limit negative publicity around security incidents, a robust notification timeline within an SLA can help manage expectations and mitigate potential harm to all involved.
Data breach notification times can vary wildly depending on where you are. Some places require companies to tell people about a breach within 72 hours, but others don't have a set time, which can make it tricky to know what's right.
It's surprising to learn that businesses often take a long time to realize they've had a data breach. Research shows they wait an average of 206 days to find out about a breach, and then another 73 days to get it under control. This delay can obviously make meeting notification deadlines difficult.
The cost of being slow to notify people can be huge. Companies that tell people within 30 days can save up to $1.5 million compared to those who take longer. This shows that being fast matters a lot.
It's interesting that a lot of people want to know about a data breach very quickly. A study from 2023 showed that 77% of people expect to be told within 24 hours, but many companies struggle to do that. This gap between what people want and what happens is worth considering.
The GDPR, a privacy law in Europe, says companies have to report data breaches to the government within 72 hours. But if they don't, the fines can be really big – up to 20 million or 4% of their worldwide revenue. That's a pretty strong motivation to comply!
Despite these rules, it's still a struggle for companies to figure out when and how to tell people about a breach. It's been reported that as many as 82% of organizations have trouble with this part, showing it's a persistent issue.
How a company tells people about a breach can impact how people see them. Personalized emails seem to be better received than generic online posts, suggesting that thoughtful communication makes a difference.
Breaches that involve sensitive medical information are particularly urgent. Healthcare organizations that don't tell people within the first 24 hours often experience a big drop in reputation and trust. That really shows how time-sensitive these situations can be.
Not all data breaches have the same notification timelines. For example, breaches of encrypted data might have different rules. It's essential to understand these complexities to follow the laws correctly.
Finally, studies show that telling people about security measures regularly, not just after a breach, can build trust over time. This proactive approach helps create a more positive relationship with customers and strengthens their security posture.
7 Key Metrics to Include in Your Software Service Level Agreement for 2025 - API request rate limits and throttling thresholds
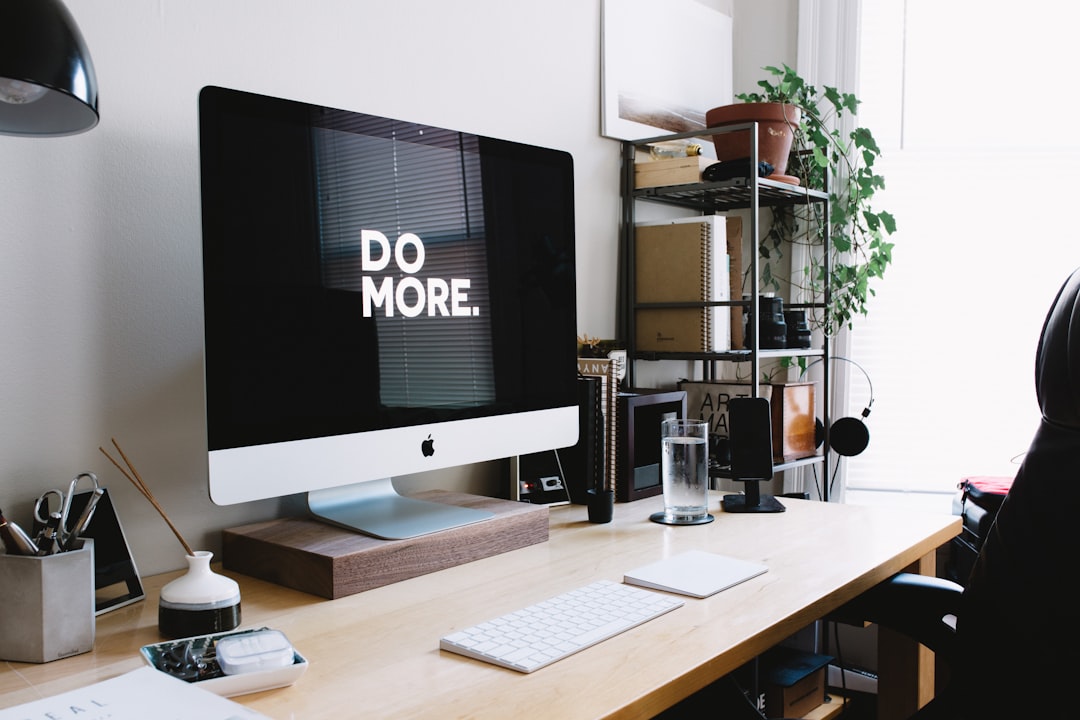
Within the context of a service level agreement (SLA), understanding how API requests are managed is vital for ensuring service quality and user experience. API request rate limits and throttling thresholds are mechanisms designed to protect server resources and ensure fairness among users by controlling the frequency of requests. Rate limiting sets a hard cap on the number of requests allowed within a specific timeframe, preventing server overload. Throttling, on the other hand, is a more nuanced approach that dynamically adjusts the speed of requests, slowing down traffic when needed instead of abruptly blocking it. These limits and thresholds are often tied to user accounts or specific application identities, demonstrating a layered approach to access control.
While limiting request rates might seem restrictive, it's actually crucial for maintaining service stability. For example, an excessive number of requests to a particular API endpoint could overwhelm a server, leading to delays or outright failures for other users. This is where the HTTP 429 error code becomes relevant, signaling that a user has reached their allocated limit. These errors, while necessary from a technical standpoint, can frustrate users if not handled properly, making it important for providers to communicate them effectively. Monitoring and understanding the frequency and patterns of rate limit hits can help developers fine-tune their strategies for managing requests, optimizing server performance, and ensuring a positive user experience. Ideally, a well-defined SLA would include details about these rate limits and thresholds, providing clarity for both providers and users. However, this area can be tricky, as what works for one service may not translate well to another. There's always a tradeoff between stringent controls and the ease of service use, so flexibility is often built into the system to avoid a rigid and overly restrictive environment for common users.
API request rate limits and throttling thresholds are fascinating mechanisms designed to protect server resources and ensure fair access for all users. While seemingly straightforward, these limits introduce a layer of complexity that can be surprising.
For instance, some APIs utilize dynamic rate limiting, adjusting the thresholds in response to real-time conditions. This approach offers a degree of flexibility but can lead to unpredictability for developers who rely on consistent access patterns. Furthermore, APIs often tailor limits based on user roles or subscriptions, potentially creating a tiered system where certain users have preferential access. While understandable from a business perspective, this approach raises questions regarding the fairness and transparency of access.
Interestingly, studies suggest that clear and open documentation about rate limits can positively impact developer behavior. When developers understand the constraints, they're more likely to optimize their API usage, leading to a smoother experience for everyone. However, exceeding these limits can trigger penalties, such as temporary account bans or error messages, causing potential frustrations for developers whose applications are suddenly unavailable.
The implementation of throttling mechanisms varies widely across APIs, with techniques like token buckets and leaky buckets being used to control request frequencies. Understanding these variations is crucial for developers who need to optimize their interactions with specific APIs. Rate limits can be particularly challenging in distributed systems, where each component might have its own independent limit. The cumulative effect of such individual limits can create unexpected throttling, impacting overall application performance and potentially complicating integration efforts.
Instead of per-minute limits, some organizations favor long-term throttling with daily, weekly, or monthly quotas. This can create predictable usage patterns, but it also demands close attention to prevent sudden spikes in usage that might violate the limits. While resource management is critical, it's also worth acknowledging that stringent rate limits could potentially impede innovation. Developers might be hesitant to explore new features or integrations when faced with rigid constraints, potentially limiting the potential for creativity and novel applications.
Tools that automatically manage request rates can be very useful in minimizing developer burden by pacing requests to avoid exceeding limits. However, these tools can also mask the underlying complexity of API usage, making it more challenging to understand actual usage patterns. Finally, strict rate limits often place a greater burden on support teams, as they face an increase in inquiries from users encountering unexpected limitations or error messages. Clear communication and well-structured documentation are crucial in minimizing such issues and improving user satisfaction. The study of rate limiting and throttling really highlights the tension between protecting server resources and fostering innovation, showing that there isn't one easy answer to this complex interaction.
7 Key Metrics to Include in Your Software Service Level Agreement for 2025 - Disaster recovery time objective (RTO)
The Disaster Recovery Time Objective (RTO) defines the longest acceptable period a system or application can be unavailable after a disruption. It's a key component of disaster recovery and business continuity plans, guiding how fast an organization needs to restore operations to limit financial and operational harm. In our increasingly digital world, RTO is becoming essential for software service level agreements (SLAs) in 2025, alongside other metrics for data protection and incident response. By agreeing on an acceptable RTO, businesses clarify their recovery plans, show their risk tolerance, and build strong relationships with their service providers. In a time when downtime can severely affect both productivity and user trust, clearly defined and enforced RTOs become a fundamental element of a strong service relationship. It's a metric that can no longer be overlooked.
Disaster recovery time objective (RTO) is a fascinating concept that's crucial for understanding how organizations handle disruptions to their systems. It represents the maximum amount of time a system or application can be down after a disaster before it causes serious issues. This metric isn't just a single, fixed number; it actually varies depending on the importance of different systems within a company. For example, a really crucial system might have an RTO measured in minutes, while a less critical one could tolerate several hours of downtime.
It's interesting how RTO is often discussed without considering its relationship with the Recovery Point Objective (RPO). RPO is about how much data a business can afford to lose during a disaster. These two metrics go hand in hand, and both are key to creating a solid plan for recovering from a disaster.
One surprising thing about RTO is the influence of human error. It's a leading cause of downtime in most organizations, highlighting how important training and preparing people to handle problems is for keeping RTOs within reasonable bounds. And if things go wrong and the RTO isn't met, the financial hit can be substantial, with research suggesting companies can lose tens of thousands of dollars per hour of downtime.
In some industries, like finance, regulations dictate what the RTO should be, making disaster recovery a legal requirement as much as a business strategy.
Despite its importance, it's worrying that a large percentage of companies don't actually test their disaster recovery plans to see if they can meet their RTO. Running regular drills is a great way to see if your plans are realistic and to iron out any wrinkles. RTO is also part of a bigger picture, business continuity planning. Integrating RTO metrics into overall business continuity planning helps make the recovery process smoother.
Technology is advancing rapidly, and tools like cloud computing and virtualization have dramatically reduced the RTO for many companies. This means services can be restored in minutes rather than hours or days. Including RTOs in service level agreements (SLAs) provides a clear understanding for both service providers and clients of what a reasonable recovery time should be, creating accountability for meeting those goals. Lastly, setting up systems to monitor everything in real-time is a fantastic way to potentially spot problems and start the recovery process before it even affects users, which keeps RTOs low and minimizes downtime.
It's clear that understanding RTO and related factors is more important than ever. As organizations continue to depend on software and services, a well-defined and tested approach to disaster recovery is crucial.
7 Key Metrics to Include in Your Software Service Level Agreement for 2025 - Customer support ticket resolution timeframes
When crafting a software service level agreement (SLA) for 2025, it's crucial to include metrics around how quickly customer support tickets are handled. Metrics like the average time it takes to fully resolve a ticket show how efficient your support processes are. Similarly, first response time – how long it takes for a support team to acknowledge a new ticket – is a strong indicator of how responsive your service is. Keeping tabs on the number of tickets still waiting for a resolution (the ticket backlog) provides valuable insights into potential problems in your support workflows that could be slowing things down.
These timeframes are important for establishing realistic expectations for users and for identifying where improvements can be made in the support process. When support issues are handled swiftly and effectively, it boosts user satisfaction and strengthens the trust between the service provider and the end user – especially vital as software systems grow more complex. While many factors play a role in user experience, focusing on these support timeframes will contribute to building a more robust and reliable relationship with your user base.
When looking at how well customer support is performing, a few key aspects are often measured in a service level agreement (SLA). These help set expectations for both the service provider and the customer. One of the most obvious things is how long it takes to get an initial response to a support ticket. This "first response time" is a basic measure of how responsive the support team is.
Then there's the "average resolution time", which gives an idea of how long it generally takes to fully address a customer's issue. This paints a picture of how efficiently the support process is working and how satisfied customers might be. "Ticket resolution time" is a similar concept, looking at the average time it takes for the support team to resolve the tickets they get, again highlighting both how well they are doing and how effectively they handle their work.
Another way to look at it is a "customer-based SLA", which means the agreements can be unique to specific clients or groups of clients. So the terms for support could be quite different depending on who the customer is. Also important is "self-service metrics", which tracks how often customers are able to solve their problems without contacting support. This is helpful because if customers can find the answers they need on their own, the support team gets fewer tickets, showing that the support system as a whole is working better.
"Client satisfaction rate" is a direct measure of how happy customers are with the support they get. This is a crucial indicator for ongoing improvement in service delivery. Then there's the "resolution rate", which is simply the percentage of tickets that are successfully resolved. It shows the overall performance of the support team and how well the service is functioning in general.
"Ticket backlog" is also helpful for understanding how much work the support team has. It tracks the number of unresolved tickets, providing insights into the workload and efficiency of how tickets are handled. Similar to that are "help desk metrics", which includes things like call handling time and the number of tickets that are resolved. These provide useful insights into the performance of the support team and also the workload.
Finally, it's worth noting that "service level agreements" (SLAs) themselves play a key role in creating good customer experiences. When the expectations for support are clearly laid out and the service provider consistently meets them, it can foster trust and improve customer satisfaction.
It's interesting that so much emphasis is on metrics. Human error is surprisingly often the cause of delays, highlighting a need for user-friendly interfaces and training. There's a noticeable increase in resolution times when ticket volume grows. Customer expectations for speedy resolution are high, but it appears many companies don't meet them, potentially harming customer loyalty. Automating common tasks can have a positive impact, and categorizing tickets as high or low priority helps manage resources more effectively. It seems gathering feedback from customers on their experiences can also help improve support processes. Finally, multi-channel support options appear to be more effective than just one support channel, potentially offering a faster, better experience for customers. This really showcases the complex and dynamic nature of managing a quality customer support experience in an increasingly software-driven world.
More Posts from rfpgenius.pro: